Identification and patient blood subtypes
Diagnosing blood diseases often involves the identification and characterization of patient blood samples. Mathematical neural-type methods can be very useful in the automated recognition of blood cell subtypes. By having this data available, mass processing of these allow the implementation of an automatic detection model and classification of cells such as eosinophils, lymphocytes, monocytes and neutrophils. The goal of the study "Convolutional Neural Network and decision support in medical imaging: case study of the recognition of blood cell subtypes", published in the CEUR Workshop Proceedings, ISSN: 1613-0073, is to use the learning Convolutional Neural Network (CNN) type deep machine for the recognition of blood cell type images and to make them capable of classifying them such as eosinophils, lymphocytes, monocytes or neutrophils. Accuracy of classification on all learning data is 97.39% and the validation accuracy is 97.77%. Failure of image detection is very low.
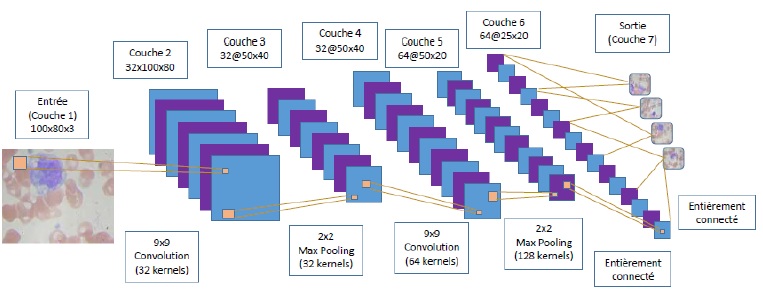
CNN Classifier is a great method for identification of blood cells.